Data Virtualization for Manufacturing Efficiency
Data virtualization is revolutionizing the manufacturing industry by seamlessly integrating data from multiple sources and presenting it in a unified, user-friendly format.
This technology enhances operational efficiency, simplifies data management, and ensures real-time access to critical information, making it an indispensable tool for modern manufacturers.
What is Data Virtualization in Manufacturing?
Data virtualization in manufacturing works like assembling different sub-assemblies into a final product. It gathers data from multiple sources and presents it in a user-friendly way. The introduction of data virtualization technology has enabled business users to seamlessly integrate data from various sources, allowing for self-service reporting and significantly accelerating the speed of business operations.
Benefits of Data Virtualization
-
Real-Time Access: Allows teams to access and analyze data from various systems instantly, enhancing decision-making and efficiency.
-
Simplifies Integration: Streamlines the process of combining data from different sources, reducing the time and effort needed for data preparation.
-
Breaks Down Silos: Provides a unified view of data across departments, promoting better collaboration and information sharing.
-
Enhances Security: Ensures secure access to sensitive data without physical replication, minimizing the risk of data breaches.
-
Scalability and Flexibility: Adapts easily to growing and changing data needs, accommodating new data sources or systems seamlessly.
Data virtualization thus enables manufacturers to operate more efficiently, securely, and flexibly, improving overall operational performance.
Challenges in Manufacturing Data Management
Vast Data Volumes: Manufacturing processes create extensive data. Efficiently managing this information is critical for success.
Varied Data Sources: Each system in a facility generates unique data, complicating integration and increasing error risk.
Isolated Data: Data often remains confined within specific departments, obstructing seamless access and collaboration.
Security Needs: Protecting sensitive manufacturing data like proprietary designs and customer details is crucial to prevent unauthorized access.
Real-World Examples of Data Virtualization Success
Many manufacturing companies have successfully implemented data virtualization to enhance their operations. For instance, a global automotive manufacturer used data virtualization to streamline supply chain management. By integrating data from suppliers, warehouses, production and data warehousing facilities, and data lakes in real time, they optimized inventory levels, reduced lead times, and improved overall supply chain efficiency.
Another example is a pharmaceutical company that utilized data virtualization to improve research and development. Researchers accessed a unified view of data from clinical trials, laboratory experiments, external sources, and integrated enterprise data. This data discovery and integration accelerated drug discovery and boosted the efficiency of their R&D efforts.
These examples highlight how data virtualization enables manufacturers to make data-driven decisions, enhance operational efficiency, and drive innovation.
Steps to Implement Data Virtualization in Manufacturing
Step 1: Assess Your Data Infrastructure
-
Review your existing data systems.
-
Identify which data sources need virtualization (e.g., manufacturing execution systems, enterprise resource planning systems, quality management systems).
Step 2: Choose the Right Data Virtualization Platform
-
Select a platform that supports real-time data integration.
-
Ensure it offers a unified view of data.
-
Verify robust security features.
Step 3: Define Architecture and Design Data Models
-
Outline the data virtualization architecture.
-
Map relationships between data sources.
-
Create virtual views accessible to users and applications.
-
It's crucial to focus on creating complete virtual data environments for software testing, development, and production support, ensuring access to high-quality data and the ability to identify causes of production issues.
Step 4: Establish Data Governance Policies
-
Implement procedures to maintain data quality, consistency, and compliance.
-
Regularly monitor and maintain the data virtualization environment for optimal performance and reliability.
Step 5: Provide Training and Support
-
Educate users on how to access and analyze data using the virtualization platform.
-
Foster a data-driven culture within the organization.
By following these steps, manufacturers can effectively implement data virtualization, leading to significant operational improvements and innovation.
Data virtualization vs. Middleware
Middleware and data virtualization tools are both essential components in the realm of data management, but they serve distinct purposes and have different functionalities.
Middleware acts as a bridge between different systems, applications, and databases, facilitating communication and data exchange between them. It primarily focuses on enabling interoperability and communication between disparate systems, often using messaging protocols or APIs. Middleware commonly handles tasks such as data transformation, routing, and integration, making it easier for different systems to work together seamlessly.
On the other hand, data virtualization tools focus on providing a unified view of data from multiple sources without the need for physical data movement or replication. Data virtualization creates a layer of abstraction that allows users to access and analyze data from various sources in real-time, without the complexities of integrating data at the physical level. This approach simplifies data access and analysis, making it easier for organizations to leverage their data assets effectively. Moreover, data virtualization offers data services that manage and integrate data from multiple sources with centralized security and governance, providing real-time access and a single virtual view of the data, contrasting with the role of middleware which does not offer such unified master data management services.
In summary, while middleware focuses on enabling communication and integration between systems, data virtualization tools focus on providing a virtualized layer for data access and analysis, including the provision of data services for a unified data view. Both play crucial roles in data management, but their functionalities and objectives differ significantly.
The Cost of Ignoring Data Virtualization
Ignoring data virtualization leads to significant technical debt in manufacturing. This debt results from shortcuts in developing and maintaining software systems, negatively affecting data management efficiency.
Without data virtualization solutions, organizations depend on manual data integration. This process is time-consuming and prone to errors, causing data quality inconsistencies. Consequently, decision-making is hindered and requires constant manual intervention.
Data silos also form without data virtualization. Information becomes segregated across departments or systems, making it difficult to access a unified data view. This fragmentation hampers collaboration and leads to duplicated efforts and redundant data storage. Over time, managing these silos increases complexity and reduces agility.
The inefficiencies and risks associated with managing data stored in disparate source systems without leveraging data virtualization are significant. It becomes challenging to ensure data accuracy and security, leading increasingly complex data leads to potential data breaches and compliance issues.
The lack of data virtualization makes it hard to adapt to changing data requirements or integrate new data sources. This inflexibility results in outdated information and missed opportunities to leverage emerging technologies, compounding technical debt.
In summary, not utilizing data virtualization in manufacturing results in various forms of technical debt. Embracing data virtualization mitigates these issues, streamlines data management, and unlocks the full potential of data assets.
AI's Role in Data Virtualization
Artificial Intelligence (AI) significantly enhances the data virtualization capabilities in manufacturing. AI algorithms automate data integration, reducing manual effort and ensuring efficient data handling. AI identifies patterns and insights from large data volumes, enabling predictive analytics and proactive decision-making. This allows organizations to optimize operations based on real-time data analysis.
AI-driven data virtualization improves access to data delivery and analysis for data consumers, enabling better decision-making. These data consumers, ranging from end users to stakeholders in various sectors, benefit from quick and efficient access to data for analytics, decision-making, software testing, and real-time delivery, emphasizing the importance of governance, security, and collaboration among them.
AI also enhances data security within virtualization frameworks. AI algorithms detect and prevent security threats such as unauthorized access, and safeguarding sensitive manufacturing data. AI-driven tools generate advanced data visualizations and reports, making it easier for teams to interpret increasingly complex data. This deeper insight facilitates informed decision-making. In conclusion, AI improves data virtualization through better automation, security, and analytics, allowing manufacturing organizations to fully harness their data and drive efficiency, innovation, and strategic decision-making.
Data Virtualization FAQs
What Is Data Virtualization?
Data virtualization is a technology that allows for real-time or near-real-time access to data from multiple sources through a data silo virtualization layer. This approach enables users to access and manage data without physically consolidating it, providing a unified and agile data management solution.
Why Do We Need Data Virtualization?
Data virtualization is needed to quickly integrate data from diverse sources, reducing the time and complexity associated with traditional data extraction and loading processes. It facilitates faster decision-making and enhanced agility in responding to changing business requirements.
What Is the Difference Between Data Warehouse and Data Visualization?
A Data Warehouse is a centralized repository designed for query and analysis, storing integrated data from multiple sources. Data Visualization refers to the graphical representation of information and data, enabling users to see analytics presented visually, which helps to understand trends and patterns.
What Is the Difference Between Data Virtualization and ETL?
ETL (Extract, Transform, Load) involves extracting data from different sources, transforming it to fit operational needs, and loading it into a database for analysis. Data Virtualization bypasses this physical process by providing an integrated view of data in real-time, without moving or transforming data in traditional ways.
What Is the Difference Between Data Lake and Data Visualization?
Data Lake is a storage architecture that holds a vast amount of raw data in its native format until it is needed. Data Visualization is the practice of converting data into a graphical format to facilitate clearer communication of trends and outliers, which is unrelated to how data is stored.
What Is the Difference Between Data Federation and Data Consolidation?
Data Federation integrates disparate data sources into a virtual database where queries can be run without physically moving data. Data Consolidation involves physically bringing multiple heterogeneous data sources together into a single storage location, typically within a data warehouse or a database.
What Is the Difference Between Data Integration and Data Federation?
Data Integration involves combining data from different sources into a single, unified view. This process usually involves physical data movement. Data Federation is a subset of data integration that provides a consolidated view of data from multiple sources without actually moving the data, relying instead on a virtual layer.
What Is Data Virtualization Software?
Data virtualization software creates a virtual layer that accesses, manages, and retrieves data without requiring technical details about the data, such as how it is formatted or where it is physically located. This software facilitates the real-time or near-real-time availability of data, helping organizations to streamline operations and improve decision-making processes.
Share this
You May Also Like
These Related Stories
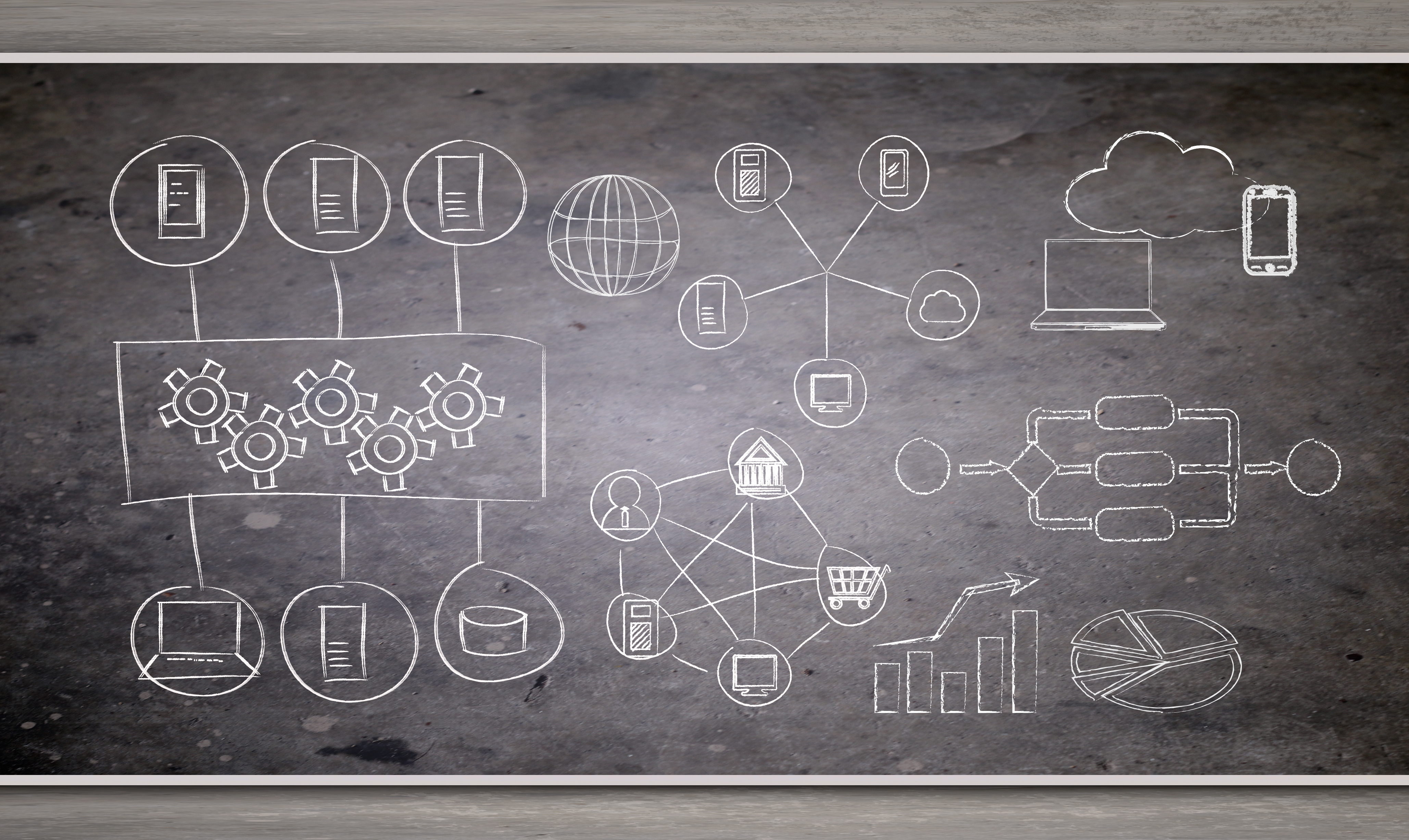
Best Practices for Manufacturing Systems Integration
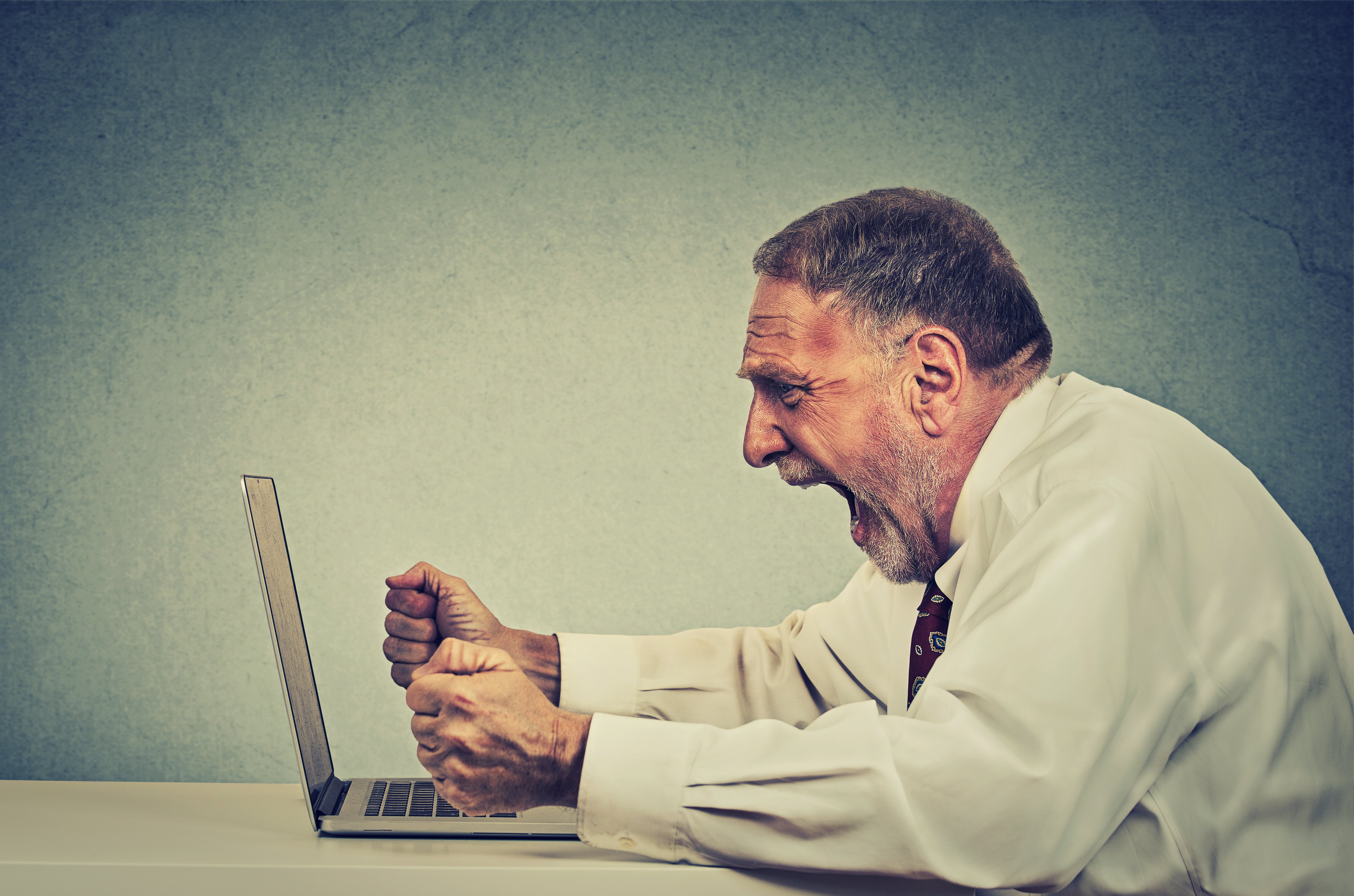
Why manufacturers stop using software after successful implementation?
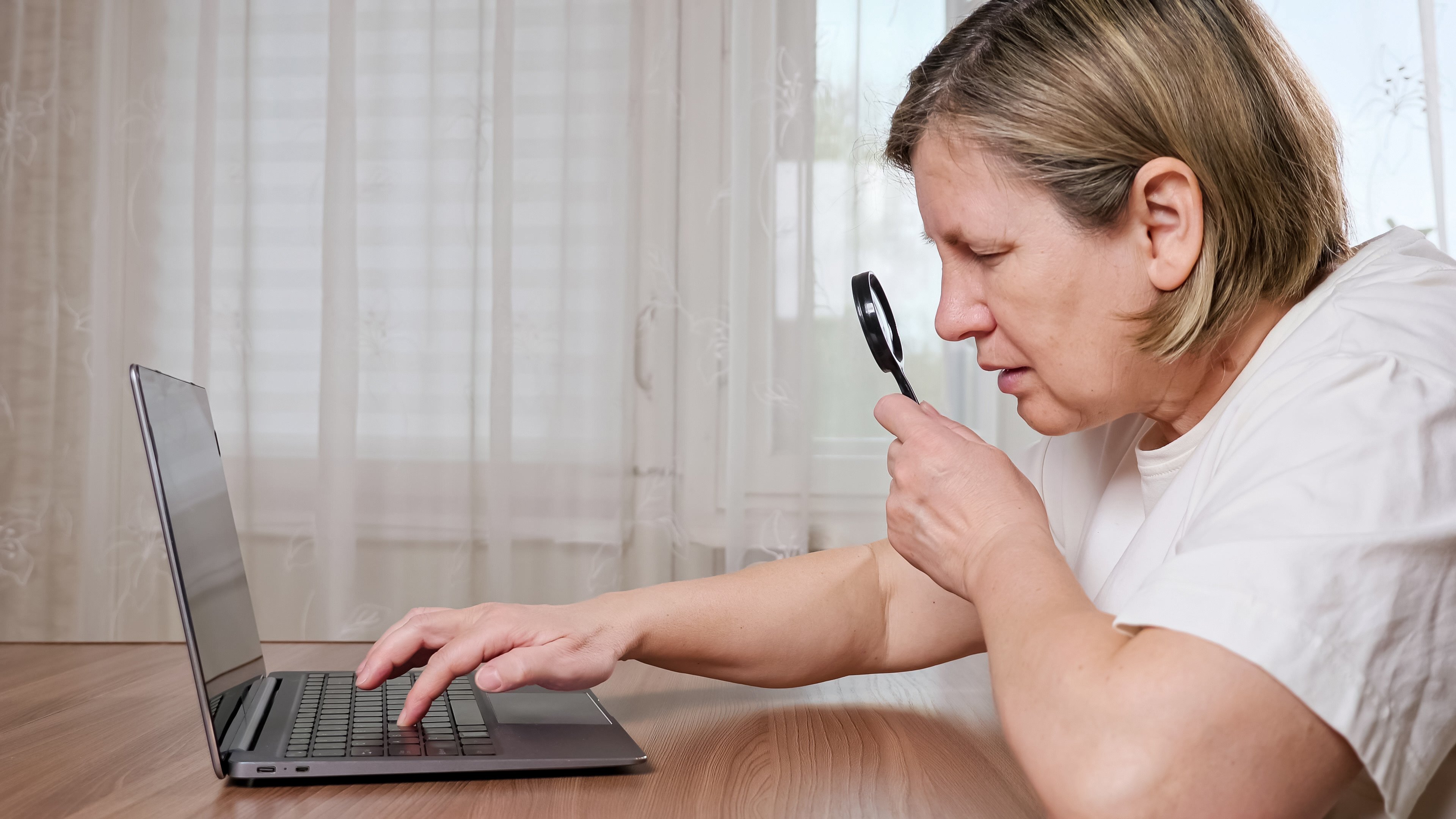
No Comments Yet
Let us know what you think