Industrial-Grade AI: Transforming Operational Efficiency
Industrial AI leverages artificial intelligence to improve industrial operations. It uses technologies like machine learning and robotics to optimize productivity and reduce costs.
This article explains what Industrial AI is, applications of Industrial AI, and how Industrial-Grade AI benefits manufacturers.
Key Takeaways
-
Industrial AI enhances operational efficiency and innovation by integrating advanced technologies such as robotics, machine learning, and data analytics across various industrial applications.
-
The adoption of industrial AI in manufacturing can lead to significant cost savings, improved product quality, and real-time optimization of processes, giving companies a competitive advantage.
-
Challenges to adopting industrial AI include data quality issues, the reliance on legacy systems, and the need for skilled personnel; overcoming these barriers is essential for successful implementation.
Understanding Industrial AI
Industrial AI means using AI in industrial applications. That’s goods movement, supply chain management, advanced analytics and automation. It automates, optimises and improves efficiency, solves pain points and creates customer value.
All AI, including industrial AI, is built on high quality industrial data so you get reliable predictions and good decision making. Industrial AI relies heavily on data from various sources, including sensors and process data, ensuring comprehensive insights for operational improvements. Integration complexities occur due to the multitude of heterogeneous data sources and the need for effective data management. Industrial AI governs operations in asset-intensive industries by providing robust and trustworthy results with the guardrails of engineering fundamentals. And industrial grade solutions are key to getting the most out of these technologies.
Affordable sensors, powerful computers, faster connectivity and cloud services have made it possible to deploy Industrial AI across all industrial environments. Technologies like robotics, computer vision and machine learning are key to these solutions, improving productivity through advanced analytics and real-time decision making.
These AI’s enable industrial companies to be more efficient and efficient and innovative and operationally efficient.
The Importance of Industrial AI for Modern Manufacturing
Incorporating industrial artificial intelligence into manufacturing processes has allowed companies to reduce production expenses by up to 20%. This significant cost reduction is coupled with enhanced efficiency and improved product quality, making AI an indispensable tool for modern manufacturing. Real-time data analysis through AI optimizes manufacturing processes, reduces downtime, and improves decision-making. Additionally, Industrial AI contributes to a 2 to 4 percent increase in product throughput.
Machine learning algorithms autonomously adapt manufacturing processes to process data, further boosting operational efficiency. This adaptability allows manufacturing companies to make real-time adjustments based on data analysis, ensuring optimal performance and continuous improvement.
Machine learning algorithms process data and experiences to detect patterns and make predictions, further enhancing their utility in industrial applications. However, machine learning models are often considered as black-box systems due to their complexity, which can make it challenging to interpret their decision-making processes.
The result is a competitive edge in the market, driven by superior product quality and innovative solutions. Explore the latest machine learning applications that are transforming manufacturing.
Key Applications of Industrial AI
Industrial AI applications are vast and varied, ranging from quality control to supply chain optimization. AI-driven solutions enhance quality control procedures, detecting defects more accurately than manual inspections.
Key applications include digital twins and predictive maintenance, AI-enabled robotics in warehousing, and generative AI for process optimization, each offering unique benefits and improvements in operational efficiency. Industrial AI captures relationships in large datasets, enabling predictive maintenance, quality control, and process optimization.
Digital Twins and Predictive Maintenance
Digital twins provide a virtual representation of physical systems, enabling real-time performance monitoring and optimization. This technology allows manufacturers to test new factory designs in a virtual environment before implementation, reducing risks and costs.
Additionally, digital twins allow businesses to simulate and verify industrial AI models in real-time environments before deployment, ensuring accuracy and reliability. NVIDIA Omniverse, Isaac, and Metropolis provide tools for creating industrial digital twins. AI builds digital twins to simulate and optimize production processes in a virtual environment.
Predictive maintenance, powered by advanced AI applications, forecasts machinery failures, significantly reducing unexpected downtime and scheduling maintenance activities during non-peak hours using simulation models. Industrial AI can reduce unplanned downtime by 20 to 50 percent, showcasing its effectiveness in maintaining operational continuity and efficiency. AI helps organizations analyze vast amounts of industrial data to prevent unplanned downtime through predictive analytics.
Foreseeing potential issues and preventing unplanned downtime, industrial AI solutions add tangible value to manufacturing operations. The integration of sensor readings and real-time monitoring ensures that equipment failures are addressed promptly, enhancing overall efficiency and reliability.
AI Robotics in Warehousing
AI robotics is changing warehousing by optimising supply chain operations and coordinating tasks between robots and human workers.
These collaborative robots (cobots) make it safer and more productive by doing physically demanding tasks alongside human workers. AI allows robots to handle complex and difficult to handle materials like wet and squished chicken wings, showing how versatile and adaptable these technologies are.
By integrating AI algorithms these autonomous vehicles can quickly identify and execute tasks, making it more efficient and less work for humans. The symbiotic relationship between robots and humans makes for a smoother and more efficient warehousing operation, resulting in big cost savings and operational benefits.
Generative AI for Process Optimisation
Generative AI is a powerful tool for process optimisation, it can quickly evaluate multiple design iterations and reduce product development time by 80%. By finding reuse opportunities in complex product designs AI reduces costs and shortens development cycles. In a metals manufacturing plant AI scheduling agents reduced yield losses by 20-40% showing the impact of AI on operational efficiency. Purpose-built applications in industrial AI incorporate predictive and generative AI for operational efficiency. Industrial AI integrates generative AI and AI agents with robotics, IoT, and advanced analytics.
AI considers multiple variables to find the optimal solution for manufacturing line scheduling, it uses genetic algorithms and Bayesian optimisers to improve the optimisation process. This ai approach to advanced analytics leads to better performance, increased productivity and better decision making across various industrial processes.
Industrial AI Challenges
Industrial AI is not without its challenges. Here are some of the key ones:
-
Data quality issues (inaccurate or incomplete data) can kill AI models. Data quality issues arise from noise in sensor readings and inconsistencies in data acquisition.
-
Many organisations are stuck with legacy systems that make it hard to integrate AI with existing IT infrastructure.
-
Financial barriers (big upfront costs) are a major adoption blocker.
Creating pilot projects that show the value of AI can help decision makers overcome their fears of big investments. And the shortage of skilled people (data scientists and AI engineers) is a major barrier to successful AI deployment. Many executives remain unsure where to apply AI solutions to capture real bottom-line impact. Cultural resistance and a conservative approach to new technology also slows down AI adoption in industrial environments.
Having a data driven culture that trusts AI algorithms and predictions is key to industrial AI adoption. Fostering a culture of innovation and investing in training and development will help organisations overcome the challenges and get the most out of AI.
Future Trends in Industrial AI
The future of industrial artificial intelligence is incredibly promising, with the concept of ‘smart factories’ leading the way. These highly automated and adaptable manufacturing systems integrate AI technologies to optimize operations and enhance decision-making capabilities.
The future of Industrial AI will be powered by pervasive real-time access to data, rich domain knowledge capture, and automation, driving unprecedented advancements in industrial processes. Industrial AI combines artificial intelligence with domain expertise to enhance asset-intensive industries. AI algorithms are evolving to provide increased autonomy in industrial processes, enabling more efficient and effective decision-making.
Notably, 79% of companies have an Industrial AI project live or in pilot stage. The implementation of Industrial AI can reshape market leadership across various sectors, positioning companies that adopt it early as industry leaders.
One significant trend is the development of autonomous vehicles, which aim to optimize transportation and logistics in industrial settings. As AI technologies continue to advance, new capabilities will emerge, driving innovation and transformation in the industrial sector.
The years ahead promise exciting developments that will redefine the landscape of industrial automation and operational excellence.
Case Studies: Success Stories in Industrial AI
Real-world examples show the power of industrial artificial intelligence and digital transformation. Delta Electronics reduced downtime and improved efficiency with a digital twin. Siemens Electronics Factory saw 30% productivity increase with a Copilot for Operations. AI can dramatically speed up the process of understanding root causes of product issues, further showcasing its transformative potential.
AI solutions like ContiVerse give real-time insights into factory processes, speed up implementation and navigate complexity. In the semiconductor industry one customer saved $1M in energy and 10,000 tons of CO2 per year with an AI solution.
These examples show the operational benefits and capabilities of human operators with AI.
AI in Supply Chain Management
Industrial artificial intelligence is key to supply chain management. Analyzing market data with AI systems gives accurate product demand forecasting, better efficiency and decision making. AI with Internet of Things (IoT) enables real-time data processing and analysis, better decision making in industrial environments. Implementing Industrial AI leads to increased energy efficiency by 3 to 5 percent, further enhancing its value in optimizing industrial operations.
Companies using AI for supply chain management have seen significant cost savings, 15% reduction in logistics costs and 35% in inventory levels. These benefits show the competitive advantage and efficiency AI brings to supply chain operations, driving operational excellence.
Enhancing Energy Efficiency with Industrial AI
In industry, energy efficiency is key as production can consume up to 50% of a site’s energy. Industrial AI helps sites reduce energy consumption by optimising production and resulting in big energy savings. Predictive analytics allows industries to monitor energy consumption and identify inefficiencies and get actionable insights for energy optimisation.
Tools like the EcoStruxure Industrial Advisor – Predictive Energy can reduce energy consumption by up to 10% and carbon emissions by as much as 40% and save costs. These AI driven solutions not only improve energy efficiency but also operational excellence and sustainability in industrial processes and help predict performance.
Summary
Industrial AI is transforming the industrial world by improving efficiency, reducing costs and driving innovation. From digital twins and predictive maintenance to AI enabled robotics and generative AI the applications of AI in industry are endless and varied. These technologies bring many benefits including better decision making, less downtime and better product quality.
As we look to the future the evolution of AI technologies will bring even more advancements in industrial automation and operational excellence. By overcoming adoption challenges and creating a culture of innovation industrial organisations can unlock the full potential of AI. The future of industrial AI is bright and its impact on industry will be big and wide.
Frequently Asked Questions
What is Industrial AI?
Industrial AI entails the implementation of artificial intelligence technologies within various industrial contexts, including supply chain management, automation, and advanced analytics. It facilitates enhanced efficiency and decision-making in industrial operations. Companies can teach AI to navigate structured and unstructured technical documents for knowledge discovery, further expanding its applications.
How does AI improve manufacturing processes?
AI improves manufacturing processes by facilitating real-time data analysis, which optimizes efficiency and minimizes downtime. This leads to more streamlined operations and increased productivity.
What are digital twins and how do they benefit industries?
Digital twins are virtual representations of physical systems that enable real-time monitoring and optimization, leading to improved predictive maintenance and reduced downtime in various industries. This innovative approach enhances operational efficiency and informed decision-making.
What challenges do companies face in adopting Industrial AI?
Companies face significant challenges in adopting Industrial AI, including data quality issues, integration with legacy systems, financial constraints, a shortage of skilled personnel, and cultural resistance. Addressing these obstacles is crucial for successful implementation.
How does AI enhance energy efficiency in industrial settings?
AI enhances energy efficiency in industrial settings by optimizing production processes and continuously monitoring energy consumption, resulting in significant energy savings and reduced carbon emissions.
Share this
You May Also Like
These Related Stories
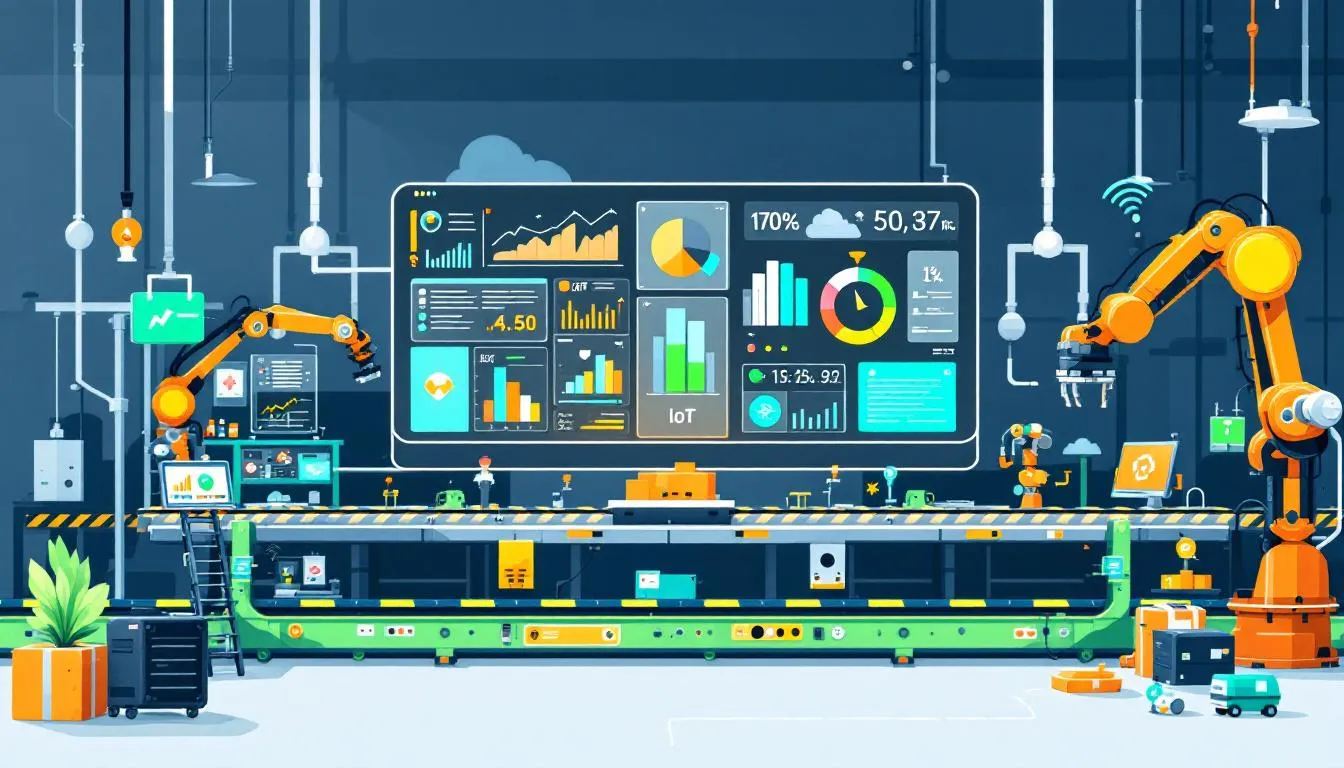
Top 7 IoT Use Cases in Manufacturing for 2025
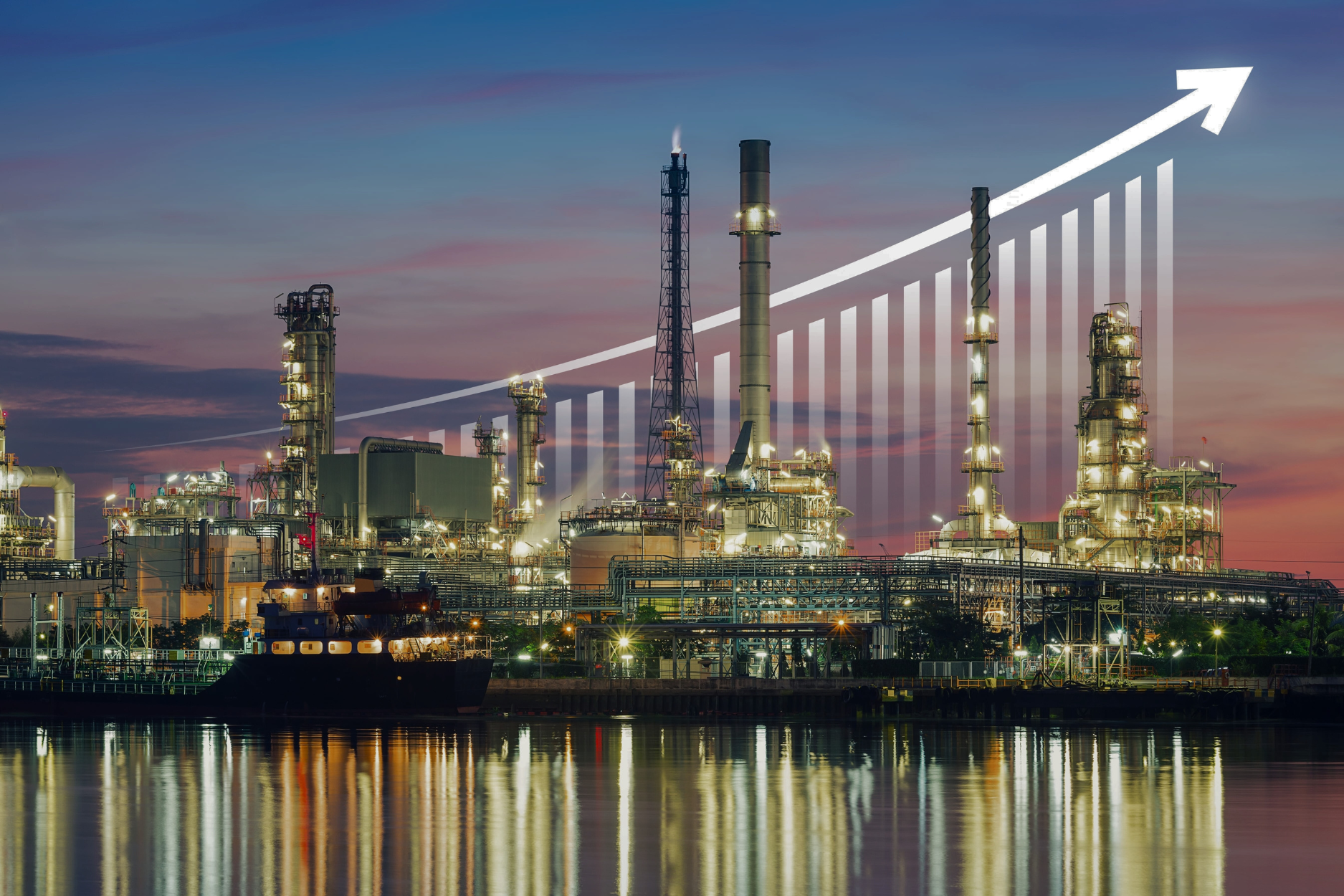
Scaling Production Processes for Small Business Manufacturers
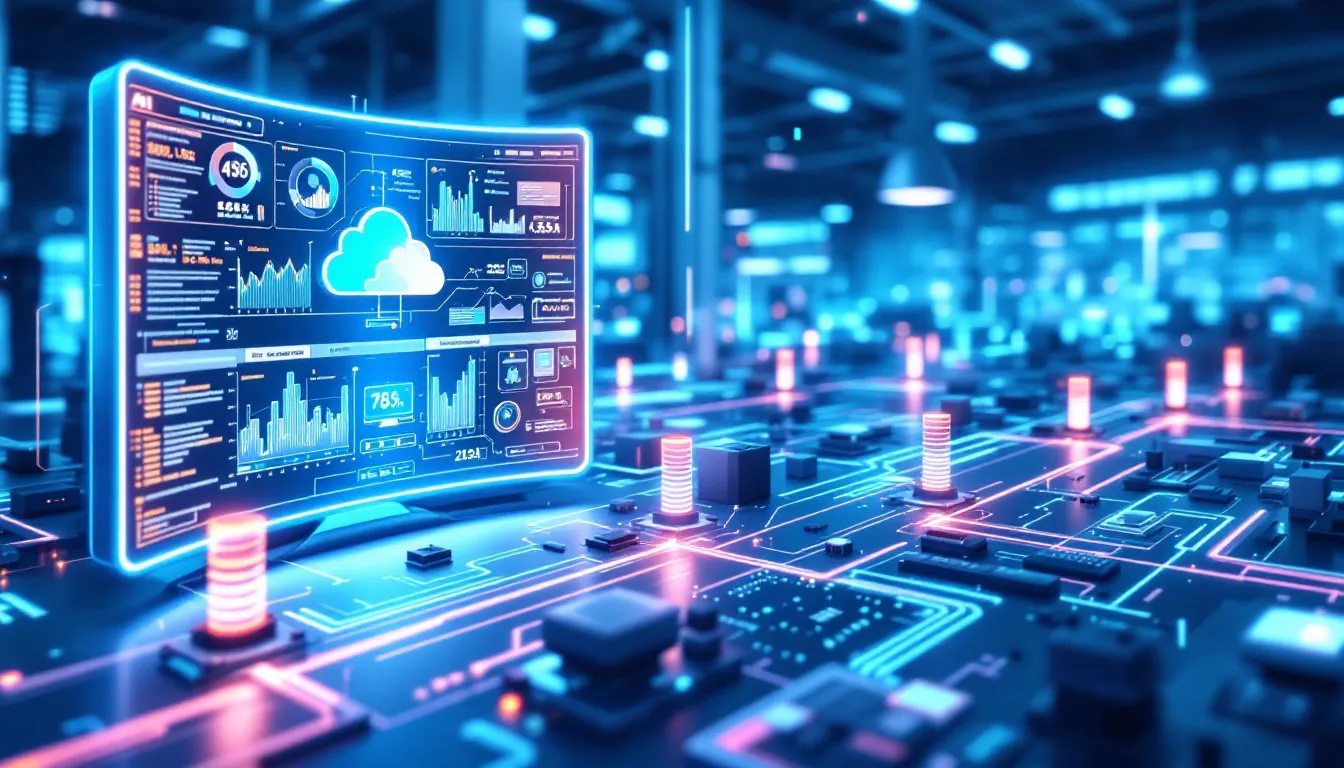
No Comments Yet
Let us know what you think